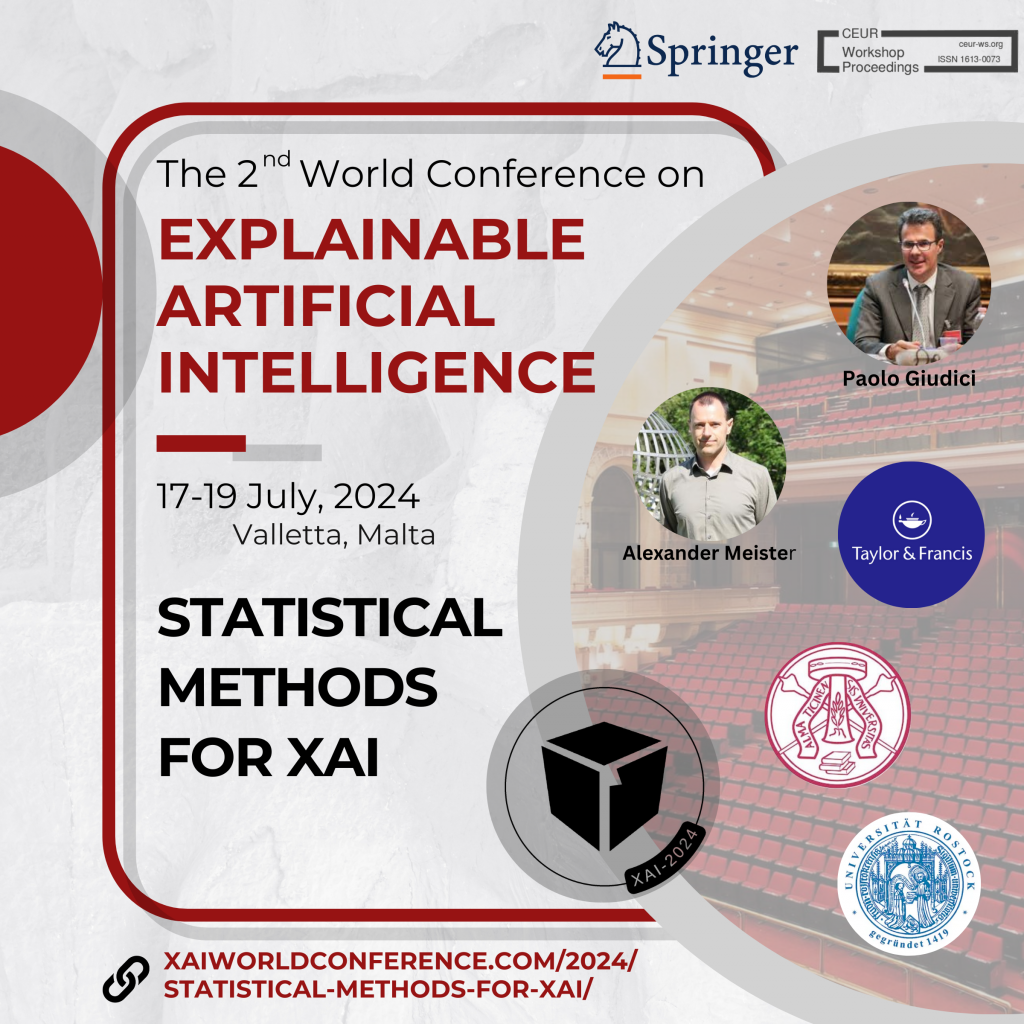
Explainable Artificial Intelligence requires appropriate statistical metrics to assess explainability and, more generally, trustworthiness of machine learning output. Examples of such metrics include measures of predictive accuracy (mean squared error, brier score, area under the curve, rank graduation accuracy) and related statistical tests (exact, asymptotic, bootstrapped); measures of explainability (difference in predictions, prediction ranks, explained variance, predictive accuracy).; regularisation methods to reduce computational complexity and improve interpretability (lasso and ridge, stepwise selection, dimensionality reduction, network analysis), and related model comparison tests; measures of robustness, under data perturbations, outliers and adversarial data (sensitivity analysis, bayesian robustness, extreme value models, bayesian robustness); measures of fairness ( group based measures, conditional measures, propensity score matching, counterfactual measures). The special track is focused on the above issues, but also their practical application in different application settings, such as automotive, finance, health and robotics. More generally, It aims at providing statistically sound solutions to the above problems, to enhance AI risk management, and make AI applications more responsible. The track is jointly organised by the two editors of the journal “Statistics” of Taylor and Francis. The Journal will organise, rightly after the conference, a special issue that can include the extended versions of the papers selected at the conference for the special track and, more generally, all selected papers that include statistical approaches for xAI.
Topics
Statistical tests for explainability |
Explainability as difference in predictions |
Explainability as difference in predictive accuracy |
Explainability as difference in goodness of fit |
Explainability as difference in concentration |
Model regularisation to improve explainability |
Lasso, Ridge and penalisation methods for improving xAI methods |
Principal components/dimension reduction methods for xAI methods improvement |
Improving robustness of explanations |
Influence functions for xAI methods |
Sensitivity analysis for xAI methods |
Outlier detection for xAI |
Reliability analysis in/for xAI methods |
Measure of fairness based on explainability |
Group based fairness for xAI |
Conditional fairness for/with xAI methods |
Propensity score matching for explainability |
Counterfactual fairness with/for xAI methods |
Statistical tests for fairness of xAI methods |